AI Strategy & Implementation
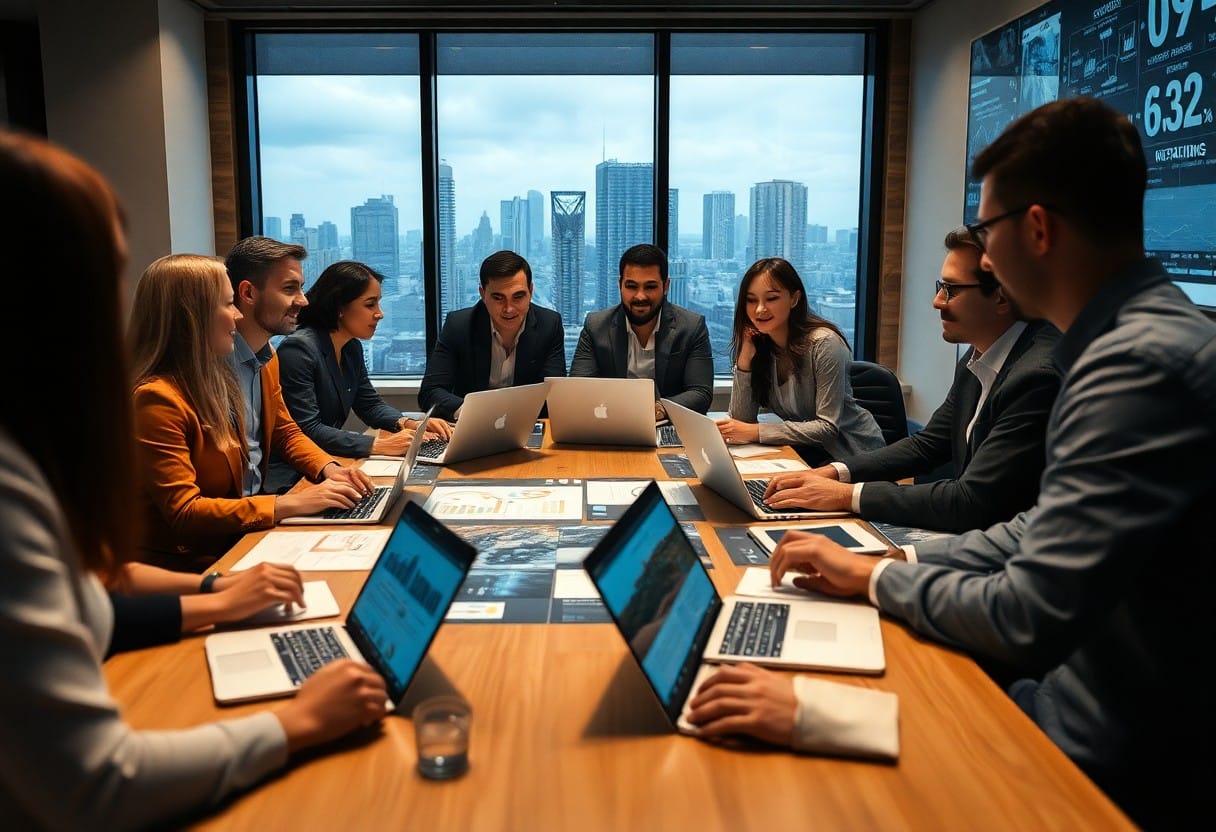
Over the past decade, AI has shifted from a futuristic concept to a vital asset in business growth. You need a well-defined AI strategy to align technology with your goals effectively. By understanding key phases—from identifying opportunities to deploying solutions—you can ensure smooth implementation and sustainable impact. This guide will equip you with important insights to drive AI adoption that enhances operational efficiency and innovation within your organization.
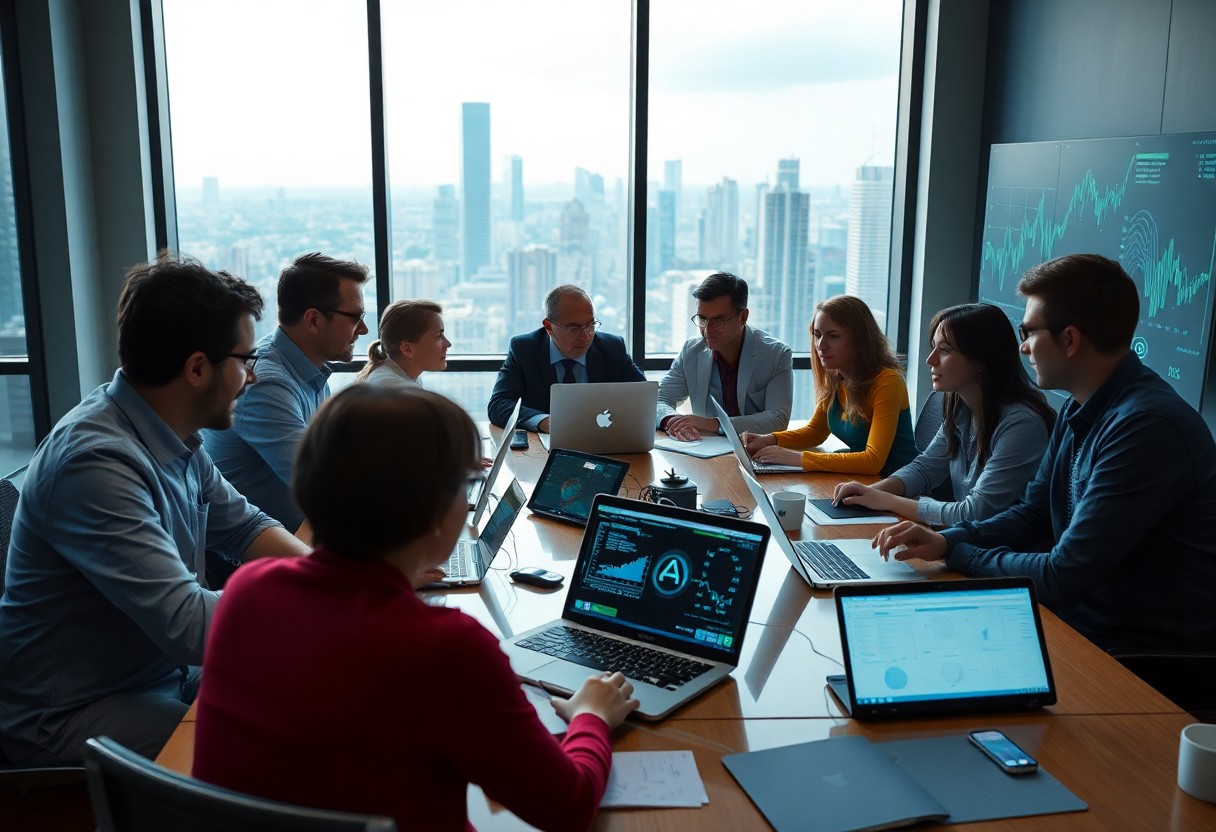
Key Takeaways:
- Develop a clear AI vision aligned with your organization's goals to ensure focused strategy and measurable outcomes.
- Invest in building cross-functional teams combining domain expertise, data science, and engineering for effective AI development and deployment.
- Prioritize data quality and availability, as well-structured data is foundational for successful AI models and insights.
- Implement scalable AI solutions with continuous monitoring and iteration to adapt to changing needs and improve performance over time.
- Address ethical considerations and establish governance frameworks to guide responsible AI use and compliance.
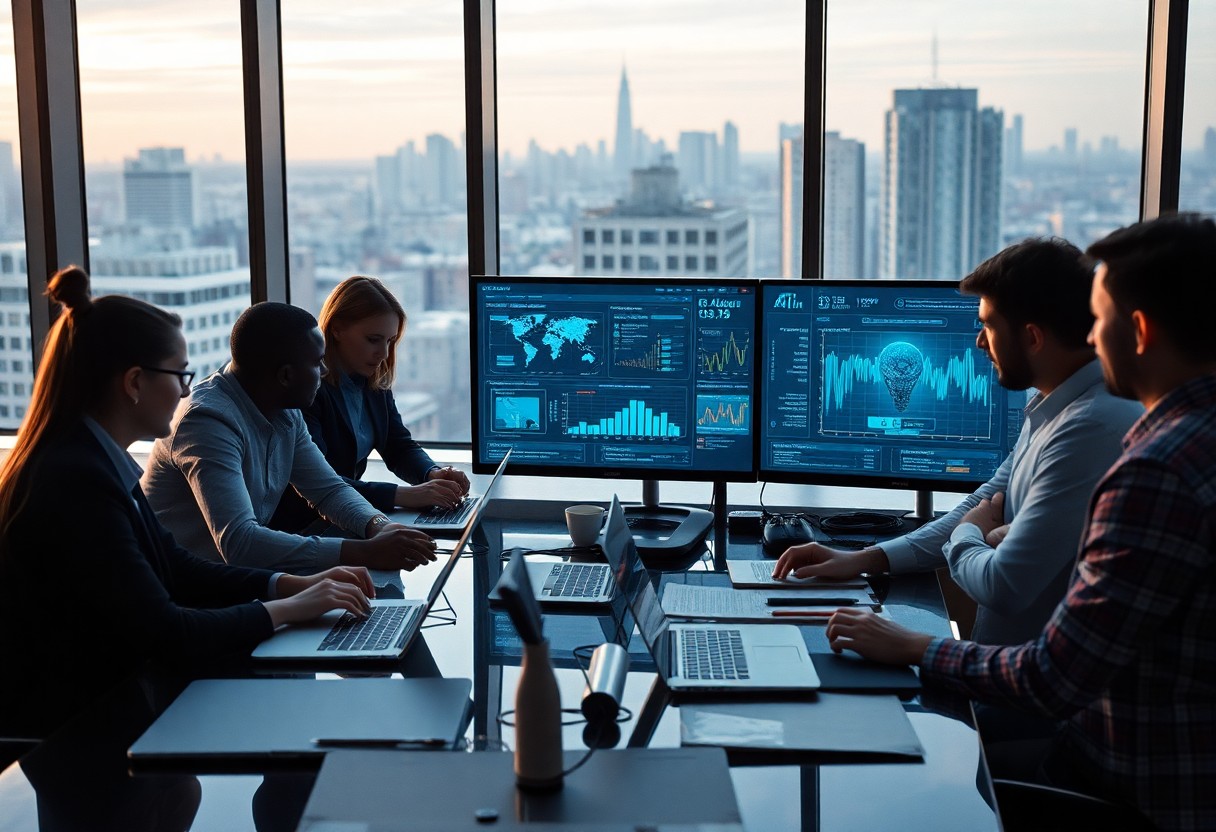
Understanding AI Strategy
Your ability to develop a clear AI strategy is fundamental to driving successful implementation and gaining competitive advantage. By defining precise objectives and aligning AI initiatives with your business goals, you create a roadmap that guides your team through complex technological transformations. For an in-depth resource, explore this Guide to AI Strategy & Implementation to strengthen your foundational understanding and approach.
Defining AI Strategy
Alongside setting your vision, defining AI strategy involves identifying where artificial intelligence can deliver the most value for your organization. You focus on prioritizing opportunities that align with your strategic goals, assessing your current capabilities, and determining the resources required to move forward effectively.
Key Components of AI Strategy
Against the backdrop of your business environment, key components of your AI strategy include governance frameworks, data infrastructure, talent acquisition, technology selection, and performance metrics. These elements work together to ensure your AI initiatives are well-organized and impactful.
Strategy requires a balanced approach: you establish ethical guidelines to govern AI use, invest in scalable data pipelines to fuel models, and build a skilled team capable of adapting to evolving technologies. Additionally, continuous monitoring and iteration allow you to refine AI applications and maximize business outcomes over time.
Developing an Effective AI Implementation Plan
Some crucial steps must be taken to develop an effective AI implementation plan that aligns with your business goals. Understanding your organization's unique needs and capabilities allows you to tailor AI initiatives for maximum impact. For more insights, explore How to Create an Effective AI Strategy. This will guide you through structuring your approach, prioritizing projects, and preparing for seamless deployment.
Assessing Organizational Readiness
Below, you need to evaluate your company's current technological infrastructure, data maturity, and staff expertise. This assessment helps identify potential gaps and areas for development, ensuring your organization is prepared to integrate AI solutions successfully. By understanding your readiness level, you can create a foundation that supports sustainable AI growth.
Setting Objectives and KPIs
Among the first actions you should take is defining clear objectives and key performance indicators (KPIs) for your AI initiatives. These metrics provide measurable targets that keep your projects aligned with overall business outcomes, allowing you to track progress and effectiveness efficiently.
Considering the importance of specific, achievable goals, you should establish KPIs that reflect value creation and operational improvements. For example, you might measure reductions in processing times, increases in customer satisfaction, or improvements in decision accuracy. Clear objectives enable continuous assessment and adjustment, helping ensure your AI strategy delivers tangible benefits.
Technology and Tools for AI Implementation
Now that you understand the strategic importance of AI, selecting the right technology and tools is necessary to transform your vision into reality. From machine learning frameworks to data processing platforms, the tools you choose will influence your AI project's scalability, efficiency, and success. By aligning your technology stack with your goals, you can streamline development while harnessing cutting-edge capabilities that propel your business forward.
Choosing the Right AI Technologies
On your journey to implement AI, you need to assess which technologies best fit your objectives. Consider the types of AI—such as natural language processing, computer vision, or predictive analytics—and evaluate their compatibility with your use cases. Balancing sophistication, ease of use, and integration capabilities ensures you invest in tools that deliver value without overwhelming your team or infrastructure.
Integrating AI with Existing Systems
Among the many challenges you will face, integrating AI with your existing IT environment requires careful planning. This involves ensuring data compatibility, maintaining system performance, and creating seamless workflows between AI solutions and legacy applications to maximize their collective potential.
At this stage, you must conduct a thorough audit of your current systems and data assets to identify integration points and potential bottlenecks. Employing APIs and middleware can simplify connections, while gradual deployment helps minimize disruptions. Prioritizing interoperability and stability will enable your AI initiatives to complement and enhance your established infrastructure effectively.
Change Management in AI Implementation
Unlike traditional technology adoption, AI implementation demands a dynamic change management approach that balances technical integration with cultural shifts. You must guide your organization through uncertainty, aligning teams behind new workflows and ethical considerations. Effective change management helps minimize resistance, maintain productivity, and foster an environment where AI initiatives are embraced rather than feared, ensuring sustained success throughout your transformation journey.
Stakeholder Engagement
Around your AI implementation, engaging stakeholders early and continuously is important. You need to communicate the benefits, potential impacts, and goals clearly to all parties—from leadership to end users. This engagement builds trust, gains buy-in, and surfaces valuable insights that refine your strategy, making your AI projects more relevant and effective across the organization.
Training and Development
For your AI initiatives to succeed, investing in training and development is vital. You should equip your teams with the skills and knowledge to leverage AI tools confidently and responsibly. Proper training fosters adaptability and empowers your workforce to contribute creatively, driving innovation alongside AI technology.
Development in training programs should focus not only on technical proficiency but also on ethical AI use and change readiness. Tailoring learning experiences to different roles helps you address specific needs while encouraging collaboration. By continuously updating these programs, you ensure your organization remains agile and capable of maximizing AI’s evolving potential.
Measuring Success of AI Initiatives
After implementing AI initiatives, assessing their impact through clear metrics and feedback loops is vital. You should define specific, quantifiable goals aligned with your business objectives, such as accuracy, efficiency gains, or customer satisfaction improvements. Regular measurement helps you verify if the AI solutions are delivering the expected value and guides adjustments needed for optimization and scaling.
Monitoring and Evaluation Techniques
Evaluation involves using both qualitative and quantitative metrics to track AI performance over time. You can employ key performance indicators (KPIs), real-time dashboards, and user feedback to gain insights. Techniques like A/B testing, scenario analysis, and error rate tracking enable you to detect deviations or emerging issues promptly, ensuring the AI remains aligned with your goals.
Continuous Improvement Practices
Below consistent refinement of AI models through iterative updates ensures sustained performance and relevance. By incorporating new data, feedback, and technological advances, you can evolve your AI applications beyond their initial deployment, adapting to changing conditions and expanding capabilities for better outcomes.
Techniques for continuous improvement include establishing automated retraining pipelines, setting up monitoring alerts for performance drops, and fostering collaboration between data scientists, domain experts, and end users. This dynamic approach empowers you to maintain high accuracy, address biases, and respond proactively to evolving business needs.
Future Trends in AI Strategy and Implementation
To stay ahead in AI strategy and implementation, you must anticipate shifting landscapes and integrate innovations proactively. As AI evolves, adopting flexible strategies allows you to leverage new opportunities and tackle emerging challenges efficiently, ensuring your initiatives remain relevant and effective in driving business growth.
Emerging Technologies
Beside advancements in machine learning, technologies like quantum computing, edge AI, and explainable AI are reshaping the AI ecosystem. By exploring these innovations, you can enhance your strategic approach and develop systems that offer improved performance, transparency, and responsiveness tailored to your organization's unique needs.
Best Practices for Adaptation
Against inevitable change, you need to cultivate agility in your AI strategies by fostering continuous learning and encouraging cross-functional collaboration. Embracing a culture that supports experimentation and feedback ensures your AI initiatives adapt smoothly to new technological and market dynamics.
Trends emphasize the importance of iterative development cycles and stakeholder engagement as you implement AI solutions. By continuously assessing results and incorporating user insights, you enhance your ability to refine processes, optimize outcomes, and align AI capabilities with your evolving business goals.
Summing up
On the whole, developing an AI strategy and executing its implementation requires you to align technology goals with your broader business objectives. You must assess your current capabilities, prioritize use cases, and invest in the right talent and infrastructure to support AI initiatives effectively. By taking a structured approach, you can ensure your AI projects deliver measurable value, drive innovation, and maintain ethical standards throughout the process. Your commitment to continuous evaluation and adaptation will empower you to stay competitive in an evolving technological landscape.
FAQ
Q: What are the initial steps to develop an effective AI strategy?
A: Developing an effective AI strategy begins with understanding the organization's business objectives and identifying areas where AI can create value. This involves assessing current data assets, infrastructure, and talent capabilities. Setting clear goals and prioritizing AI initiatives aligned with business outcomes ensures focused implementation and resource allocation.
Q: How can organizations ensure successful AI implementation across departments?
A: Successful AI implementation requires collaboration between technical teams and business units. Establishing cross-functional teams, fostering open communication, and promoting an innovation-friendly culture help integrate AI solutions. Additionally, continuous training and change management efforts support user adoption and adaptation to AI-driven processes.
Q: What role does data quality play in executing an AI strategy?
A: Data quality is fundamental to AI effectiveness, as algorithms rely on accurate, complete, and relevant data to generate insights. Ensuring data is clean, well-organized, and regularly updated minimizes errors and biases in AI models. Organizations should implement robust data governance practices and invest in proper data management tools to maintain high-quality datasets.
Q: How can companies measure the impact of their AI initiatives?
A: Measuring AI impact involves defining key performance indicators (KPIs) linked to the strategic goals set for AI projects. These KPIs might include efficiency improvements, cost reductions, revenue growth, customer satisfaction, or operational accuracy. Ongoing monitoring and evaluation help in iterating AI models and scaling successful solutions.
Q: What are common challenges faced during AI strategy implementation, and how can they be addressed?
A: Common challenges include data privacy concerns, talent shortages, technology integration hurdles, and resistance to change within the organization. Addressing these challenges involves establishing clear ethical guidelines, investing in workforce skill development, selecting compatible technology platforms, and engaging stakeholders through transparent communication and leadership support.